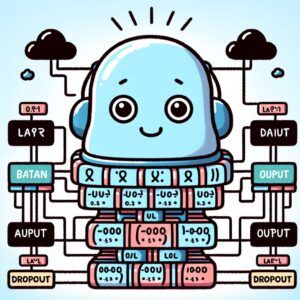
Type:
Master
UROP
Status: Available
In this thesis, the objective is to investigate the use of Monte Carlo Dropout, a Bayesian Deep Learning technique, to make uncertainty prediction on Neural Network outputs when dealing with physiological data. In particular, the student will investigate the creation of a system using Early Exit to adjust the computational power based on the uncertainty prediction.
The student will leverage state-of-the-art Deep Learning models to make predictions on publicly available datasets for health applications, e.g., ECG data from healthy and unhealthy individuals.
This project is available as a MSc thesis or as a EUROP project.
For more information contact: Leonardo Alchieri, Silvia Santini